Predicting Gentrification: Social Media Hints At Neighborhood Changes
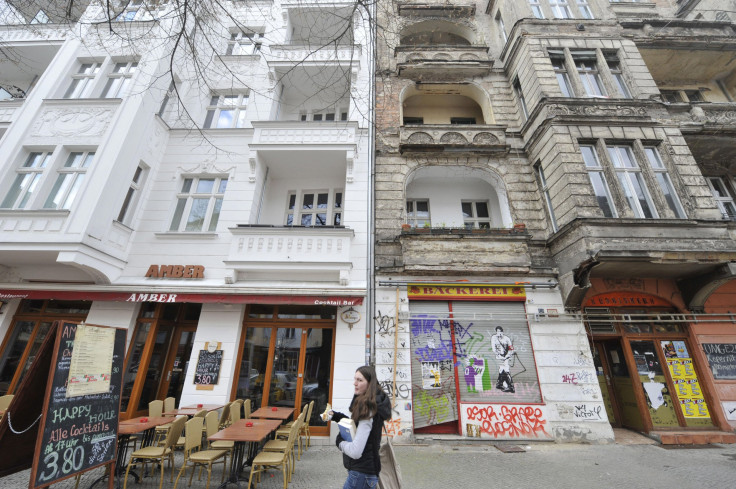
Urban planners use the term “gentrification” to describe what happens when affluent people move into a deprived neighborhood and displace existing residents. Typically, gentrification is both hard to predict and difficult to quantify. Urban dynamics are generally measured by government census data, but social media and user-generated content have made it possible to place a finger on the real-time pulse of city neighborhoods.
In fact one new study suggests predicting when a neighborhood will flip is no more than a swipe away with location-based consumer apps and social media.
A team of Cambridge researchers working with colleagues from other English universities gathered and analyzed data from an interconnected geo-social network they created based on Foursquare and Twitter. Their network model, which encompassed approximately 37,000 users and 42,000 venues in London, logged more than half a million posts and check-ins over a 10-month period. Analyzing this dataset, the research team found they could quantify social diversity of both neighborhoods and places, learning which brought together strangers as opposed to friends and which attracted new faces as opposed to regulars.
“Social ties can be classified as bridging or bonding where bonding ties are those within homogeneous groups while bridging ties are those which transcend groups and are associated with diversity,” wrote the researchers.
Hoping to measure these ties and general social diversity before analyzing gentrification, the researchers first defined four distinct features of a neighborhood: brokerage, serendipity, entropy, and homogeneity. Brokerage, the researchers say, is the ability of a community to connect people who are otherwise separated. Serendipity is the likelihood of a district bringing about chance encounters among friends. Entropy is the extent to which a place attracts diverse visitors. Finally, homogeneity is the measure of how similar visitors are in a given neighborhood.
Looking at their geo-social data, the researchers discovered that some places within a neighborhood were more likely to bring friends together while other places were better for chance encounters among strangers. For instance, a fried chicken restaurant was more likely to be the place where friends got together, while a dumpling place more often saw strangers striking up conversations. Similarly, friends were more likely to meet at a B&B, football match, or strip club, while strangers were more likely to mingle at a motel, art museum, or gay bar.
Next, the researchers compared various London boroughs based on data from the Index of Multiple Deprivation (IMD), which measures relative prosperity across England. Specifically, they compared IMD data from 2010, the year they gathered their geo-social network data, with IMD data for 2015.
Here, the researchers discovered the most socially cohesive and homogenous areas of London, the more or less static places, tended to be either very wealthy or very poor. Meanwhile, the low-income neighborhoods showing signs of increased social diversity — a variety of visitors and frequent chance encounters — had begun to undergo gentrification during the period of study.
“This suggests that affluent communities remain affluent and poor communities remain poor through isolation,” wrote Desislava Hristova, lead researcher, and her co-authors.
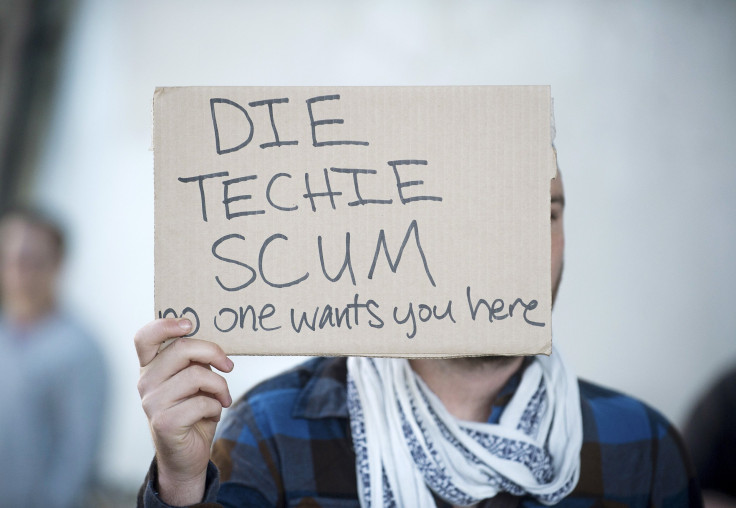
Overall, Hristova’s analysis of 32 London boroughs indicated Hackney had the highest social diversity, and in 2010, also had the second-highest deprivation, yet by 2015, this borough showed the greatest improvement on the IMD index. Today, Hackney is undergoing intense gentrification, with housing prices topping the London average, fast-decreasing crime rates, and increasing diversity of its population.
“There is a systematic feedback loop between cities and their inhabitants,” concluded the authors. Apparently, one or two brick-and-mortar truths can be found in the virtual Twittersphere.
Source: Hristova D, Williams MJ, Musolesi M, Panzarasa P, Mascolo C. Measuring Urban Social Diversity Using Interconnected Geo-Social Networks. International World Wide Web Conference. 2016.